Press notes 16/02/2022
URV researchers discover a way to predict the degradation of a neural network
The journal Nature Communications has published the results of this study led by Professor Àlex Arenas
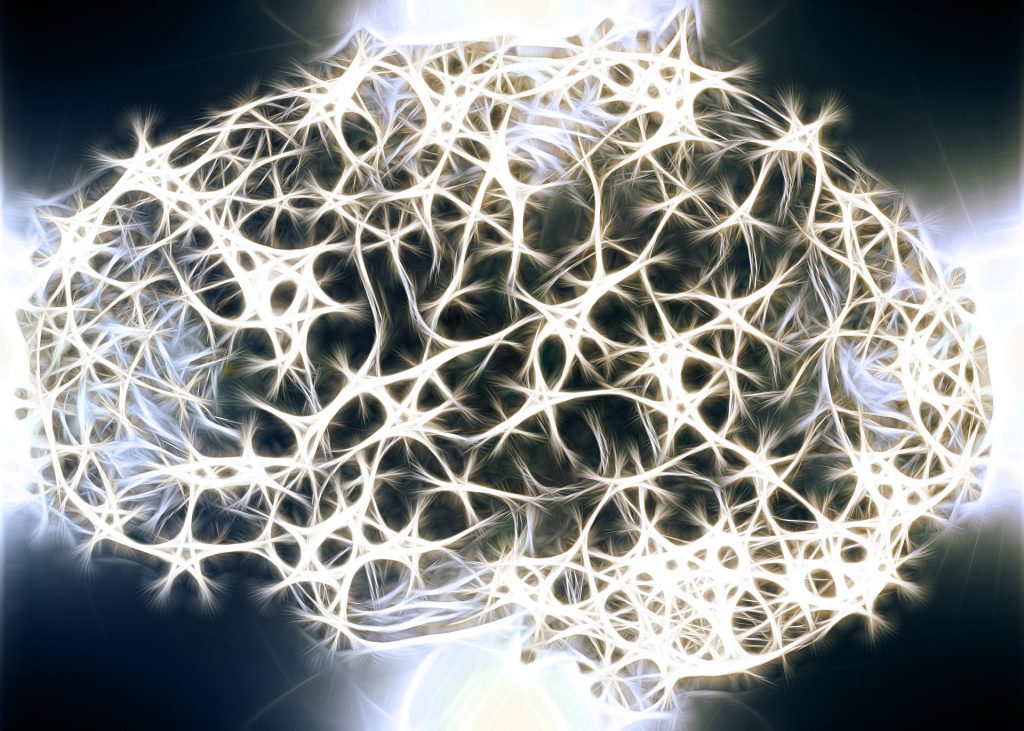
The journal Nature Communications has published the results of this study led by Professor Àlex Arenas
How does a neural network work? How does it react to a failure? How can you mathematically predicting when it will stop working and what will happen when it does. All these questions have now been answered by a research team led by Àlex Arenas, a professor at the URV’s Department of Computer Engineering and Mathematics. Arenas has found the theoretical explanation for a very complex process that will now make it possible to predict how all network system will function. The results of this research have been published in the journal Nature Communications.
Percolation is the process by which a network system suffers a failure at a particular point that ends up affecting the whole network structure. One example of this an electrical network that leaves a whole district without electricity when there is a problem in one tower. The process is even clearer in biological systems such as neurons. For various reasons, neurons degenerate until some of them die. These neuronal failures, caused by ageing, diseases or accidents, eventually lead to a significant loss of connectivity to the brain and the neural network stops working properly. The scientific community has been studying this percolation process for decades, along with what are known as phase transitions: the point at which a network will stop functioning completely if it is cut.
“Our research began a few years ago, when I was working with the UB neuroscience team led by the researcher Jordi Soriano,” explains Arenas. “We observed that, even though we directly damaged neuronal connections with lasers, the system continued to function very efficiently.” This phenomenon is known as homeostatic plasticity: despite being cut, the system tries to continue doing what it was doing before the cut. It looks for alternatives, ways to continue functioning correctly.
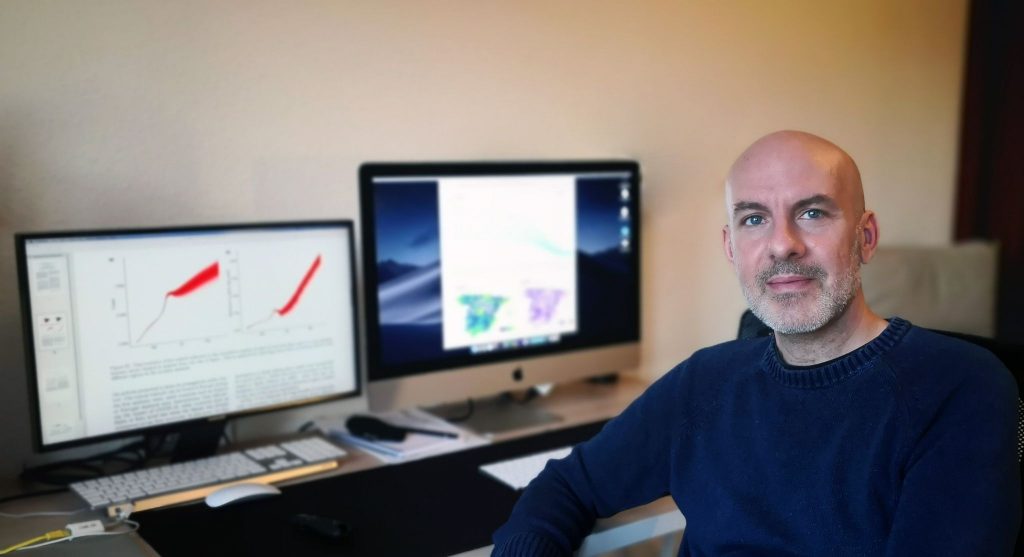
Now, the URV research team has managed to find the answers to the phase transitions of percolation degradation: that is to say, to understand how much damage a system can undergo before it will be totally degraded and lose its functionality. “We have been able to find this transition and we have also been able to calculate the homeostatic response (i.e., the ability to find alternatives and continue functioning) of the network,” says Arenas.
These results are important because the scientific community now has at its disposal a set of mathematical tools “that can be very useful not only in neuroscience but in any type of network,” he says. The research is a step forward in our understanding of how network systems react to external damage while maintaining their functionality, compensating for the failure in one of the parts and redirecting activity to another.
“Understanding these processes can provide solutions in many areas,” says Arenas, who gives as an example diseases such as Alzheimer’s, in which many patients can remember episodes from their childhood but not more recent aspects of their lives. This is related to the degradation undergone by their neural network. Now we understand why this happens and we can apply this knowledge in research in people who begin to suffer from the disease. “For example, we will be able to know how they respond to control questions, infer to what extent their neuronal system is degraded and try medication or some other sort of intervention in an attempt to reconnect, because now we know how these degradation processes act physically,” says the researcher.
The results of the research can also be applied to other fields, such as a road networks. If a road needs to be cut and traffic redirected to other areas, it will be possible to predict where there will be jams and what action will have to be taken to absorb the traffic there.
Bibliographic reference: Rapisardi, G., Kryven, I. & Arenas, A. Percolation in networks with local homeostatic plasticity. Nat Commun 13, 122 (2022). https://doi.org/10.1038/s41467-021-27736-0