06/02/2025
A URV-led study highlights the limitations of AI models in understanding language
The research compares the performance of seven AI models with that of 400 humans in comprehension tasks and reveals a lack of stability and precision in the answers
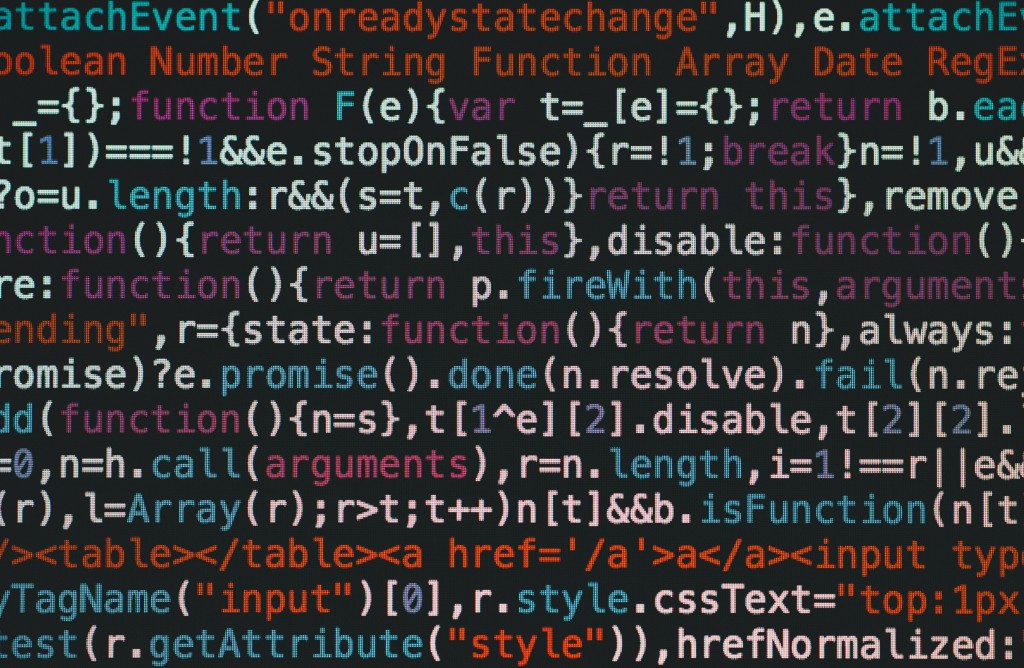
The research compares the performance of seven AI models with that of 400 humans in comprehension tasks and reveals a lack of stability and precision in the answers
An international research team led by the URV has analysed the capabilities of seven artificial intelligence (AI) models in understanding language and compared them with those of humans. The results show that, despite their success in some specific tasks, the models do not achieve a level comparable to that of humans in simple text comprehension tests. “The ability of models to carry out complex tasks does not guarantee that they are competent in simple tasks”, warned the researchers
Large language models (LLMs) are neural networks designed to generate texts autonomously from a user request. They specialise in tasks such as generating answers to general queries, translating texts, solving problems and summarising content. It is often claimed that these models have capabilities similar to those of humans, in terms of comprehension and reasoning, but the results of the research led by Vittoria Dentella, a researcher at the URV’s Language and Linguistics Research Group, show their limitations: “LLMs do not really understand language, but simply take advantage of the statistical patterns present in their training data”.
In order to compare the performance of humans and LLMs in text comprehension, the researchers put 40 questions to seven AI models (Bard, ChatGPT-3.5, ChatGPT-4, Falcon, Gemini, Llama2 and Mixtral), using simple grammatical structures and frequently used verbs. At the same time, a group of 400 people, all native English speakers, were asked the same questions and the accuracy of their answers was compared with those of the LLMs. Each question was repeated three times to assess the consistency of the answers.
The average human accuracy was 89%, far higher than that of the AI models, the best of which (ChatGPT-4) provided 83% correct answers. The results show a big difference in the performance of the text comprehension technologies: with the exception of ChatGPT-4, none of the LLMs achieved more than 70% accuracy. Humans were also more consistent when faced with repeated questions, maintaining answers in 87% of cases. In the case of the text comprehension models, on the other hand, this was between 66% and 83%
“Although LLMs can generate grammatically correct and apparently coherent texts, the results of this study suggest that, in the end, they do not understand the meaning of language in the way a human does,” explains Dentella. In reality, extended language models do not interpret meaning in the way a person does – through a combination of semantic, grammatical, pragmatic and contextual elements. They work by identifying patterns in the texts they have been given, comparing them with the patterns in the information that was used to train them and then using statistically-based predictive algorithms. Their apparent humanness is, therefore, an illusion.
The LLMs’ lack of understanding can prevent them from giving consistent answers, especially when they are subjected to repeated questions, as the study found. It also explains why the models can provide answers that are not only incorrect, but which also indicate that they have not understood the context or meaning of a concept. This in turn means, Dentella warns, that the technology is not yet reliable enough to be used in certain critical applications: “Our research shows that the ability of LLMs to carry out complex tasks does not guarantee that they are competent in simple tasks, which are often those that require a real understanding of language”.
Reference: Dentella, V., Günther, F., Murphy, E. et al. Testing AI on language comprehension tasks reveals insensitivity to underlying meaning. Sci Rep 14, 28083 (2024). https://doi.org/10.1038/s41598-024-79531-8