05/05/2023
The application of a new method of privacy protection used by the American census is unnecessary and damaging
Experts from the URV point out that this method significantly reduces the quality of the data, refuse to accept that traditional mechanisms pose a risk to privacy and call on institutions not to follow suit

Experts from the URV point out that this method significantly reduces the quality of the data, refuse to accept that traditional mechanisms pose a risk to privacy and call on institutions not to follow suit
In 2020, as it does every decade, the United States Census Bureau (USCB) published the Decennial Census, the recording of population and housing information from all fifty states, the District of Columbia, Puerto Rico and the insular areas. It is a sort of register, the results of which determine the number of seats for each state in the U.S. House of Representatives and delimit areas for distributing hundreds of billions of dollars in federal funds each year. Because the data collected is of a personal nature, the USCB has traditionally applied privacy protection mechanisms that effectively anonymised individuals and maintained the quality of the data published. However, in this edition, the USCB has implemented significant changes in the method of protection that have proved controversial. The data published by the world’s largest statistical agency mistakenly counted 5 million people twice and overlooked many others, according to an article in the New York Times.
These deviations were caused by the use of a new privacy protection method called “differential privacy”. According to researchers from the URV’s CRISES research group Josep Domingo-Ferrer and David Sánchez, this method has previously been used by large private companies, with questionable results. “There are two ways of applying differential privacy: either you do it the proper way and significantly distort the data, as the USCB has done, or you are more permissive and you conserve them, as Apple or Google have done, but then there is no guarantee of privacy,” explains Professor Sánchez.
So why would an institution with more than 120 years of history of collecting data and taking censuses use such a destructive method? Well, because the method of protection has been given a lot of publicity as a “seal of guarantee”, and in recent years it has been associated with high levels of privacy. It has attracted the attention of many researchers and professionals who use it, often “unconditionally”. Domingo-Ferrer warns that it is a method designed to deal with “certain types of data” and that it cannot be used in every situation: “It’s like cars; you wouldn’t drive a sports car on a mountain track, but it’s still a good car”.
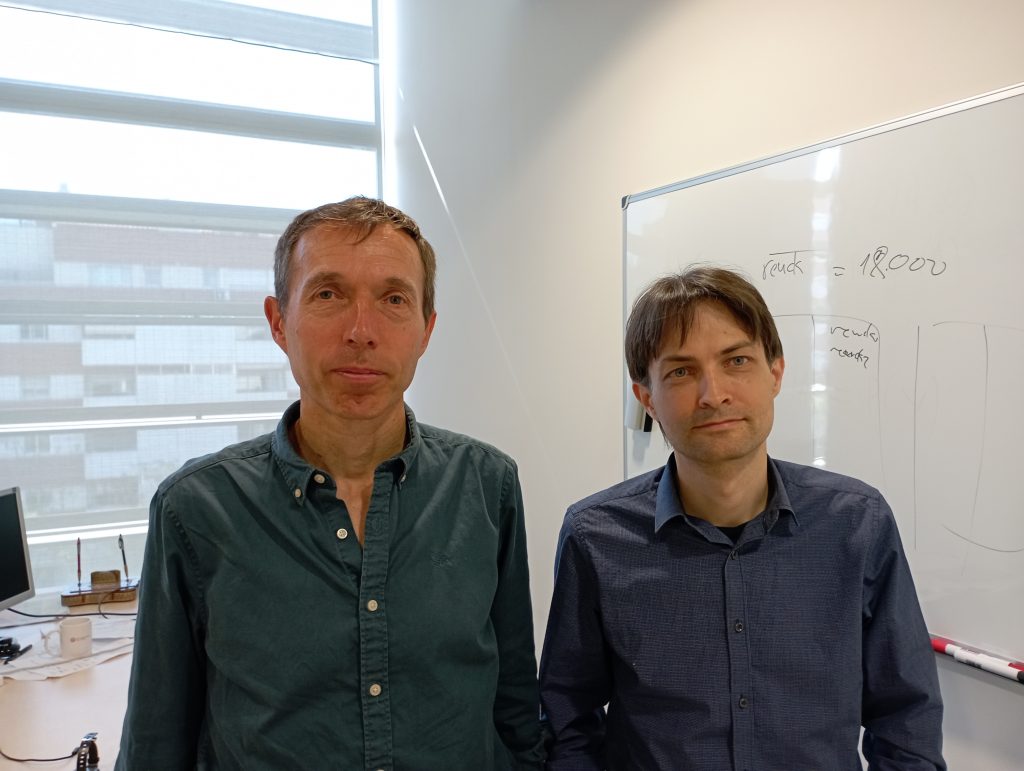
More recently, and in response to an outcry from the academic community in the United States – which has seen that the census data they need to carry out their research is much less accurate than in previous years – the creators of differential privacy published an article in the scientific journal PNAS in which they argued that, if their system was not used, the personal data of certain US citizens could be reconstructed from the data published. In their recent article in the same journal, Domingo-Ferrer and Sánchez criticise this position. “Contrary to what is stated in the original article, private information cannot be unequivocally matched to particular individuals; there are a large number of possible scenario reconstructions that are compatible with the statistics published in the census,” Domingo-Ferrer states. While it is true that by using certain statistical methods it is possible to deduce those reconstructions that are most likely to correspond to the original data, this does not necessarily involve a violation of privacy because the most likely reconstructions are those that have the most common values among the population, making it difficult to associate them with specific citizens.
As for the consequences of using differential privacy, not all of them are institutional in nature. Sánchez points out that data published by official bodies are widely used in the academic world and warns that the conclusions of subsequent research may no longer be valid or even that the research may no longer make sense. Privacy methods also play an important role in the development of machine learning technologies, which are largely trained using personal data. Researchers from the CRISES group have already shown that the use of differential privacy in this area is unnecessary and warn that it would set back the quality of artificial intelligence models significantly. “We should not give up the accuracy of these models in exchange for the label of differential privacy when there is no need,” says Sánchez.
Domingo-Ferrer and Sánchez, like many other academics in their field, are particularly concerned about institutions, especially those in European countries, being tempted to follow suit. The official statistical institutes of each country are a source of reports, censuses and other publications that are the raw material for a great deal of scientific research. “There is no imminent danger of private information being reconstructed that would justify the use of this method,” they argue. What is dangerous, they say, is if the United States Census Bureau’s approach to data protection is taken as an example. In this regard, they call on institutions and experts not to adopt such destructive methods if they are not necessary.