13/02/2023
A linguistic model enables feeling to be evaluated in social media texts
A research team from the URV has developed a system that identifies feelings in evaluative language. The results of their research have been published in the scientific journal Mathematics

A research team from the URV has developed a system that identifies feelings in evaluative language. The results of their research have been published in the scientific journal Mathematics
Words that we use in all sorts of fields on a daily basis such as fast, slow, nice, hot or normal implicitly bear an information load that is of increasing importance to companies and organisations. Evaluative language, which is used every day, makes communicative interactions interesting because it provides essential information. Sentiment analysis is an area that has received considerable attention in recent years due to the massive use of social media, which generate large amounts of evaluative text from users about all kinds of products and services. Given the interest aroused by the analysis of these texts, a research team from the URV’s Department of Romance Studies has developed a technique that includes various mathematical and linguistic methods and which can formally model evaluative statements and capture or extract the feeling (or evaluation) underlying a wide variety of linguistic expressions. The result of their research, which has been done in collaboration with the IRAFM Centre of Excellence in the Czech Republic, has been published in the scientific journal Mathematics.
To analyse feeling, computational tools are used to detect and assess evaluative language, in terms of polarity, that is: they automatically classify texts in terms of the positive or negative connotation of the language used. This analysis attempts to determine a person’s attitude to a topic. Attitude can be a judgement or evaluation, an affective state (the emotional state of the author when writing), or the emotional communicative intention (the emotional effect that the author tries to cause in the reader). In order to function, these sentiment analysis tools require formal models that can describe evaluative language in such a way that it can be processed by a machine.
Evaluative language is said to be uncertain or vague, since it is very difficult to delimit the meaning of everyday words such as good, bad, big, small, love, hate, etc. For example, a 5-year-old can be “tall” if they are 130 cm, and an adult basketball player, on the other hand, is “tall” if they are 220 cm. This variability can also be found across cultures: for example, the final meaning of the adjective “tall” is surely different in the American and Japanese conceptions. Although the final meaning is different, everyone can understand that “tall” means a high value on a height scale. A model to characterize this “fuzziness” in meaning is a diffuse model, and this is the basis of the proposal of this research, headed by Adrià Torrens and María Dolores Jiménez, of the Research Group in Mathematical Linguistics from the URV’s Department of Romance Studies, together with Vilém Novák, from the University of Ostrava in the Czech Republic.
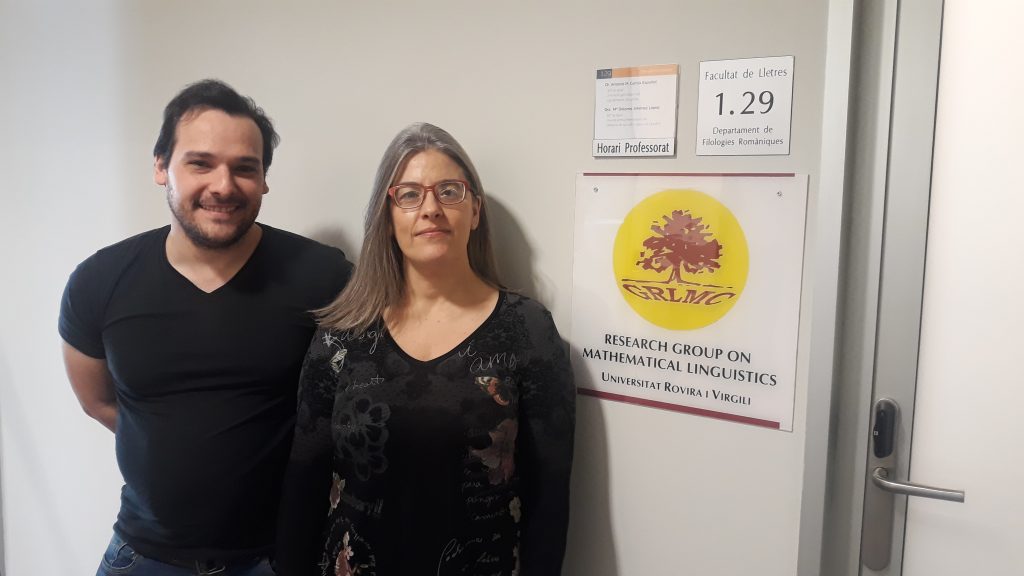
Formally modelling evaluative statements and capturing or extracting the feeling (or evaluation) behind these linguistic expressions is undoubtedly a challenge. Typically, both machine learning algorithms and dictionary techniques (known as “bag of words”) are used for these tasks.
Learning algorithms focus on aspects of computational performance. In general, these techniques do not provide sufficient features from the point of view of linguistic processes. This research aims to present a new approach based on a formal interdisciplinary model that identifies and analyses the uncertain nature and vague information of evaluative expressions, addressing many of their nuances and offering an “explanatory” idiosyncrasy.
The model proposed by this research team combines a property grammar and a fuzzy logic model. The property grammar establishes the constraints/ conditions that a linguistic structure must meet to be appropriate. The fuzzy model captures the vagueness of these expressions (“tall/high” can mean 130 cm or 220 cm), and determines the degree of positivity and/or negativity of an expression (any word can be more or less positive or negative depending on the context in which it is used). This model is expected to have numerous applications and a major impact on areas such as data mining, language learning tools, automatic authorship detection, etc.
The research does not end here. According to the research team, the next step is to carry out an interdisciplinary project with professionals in psychology, computational engineering, linguistics and lexicography to construct a set of evaluative nuclei that can be applied in sentiment analysis, similar to the WordNet project at Princeton University. “This would help to identify violent language and would also have benefits for data analysis in such service sectors as tourism. It would also help detect cognitive problems in relation to the semantic level of language,” explains María Dolores Jiménez, one of the researchers involved in this research.
Reference: Torrens-Urrutia, A., Novák, V., & Jiménez-López, M. D. (2022). Describing Linguistic Vagueness of Evaluative Expressions Using Fuzzy Natural Logic and Linguistic Constraints. Mathematics, 10 (15), 2760. https://www.mdpi.com/2227-7390/10/15/2760