15/06/2023
A mathematical model has been designed to estimate the effectiveness of drugs against viruses
Two URV research groups have created a machine learning model that may reduce the time needed to develop drugs against new viruses.
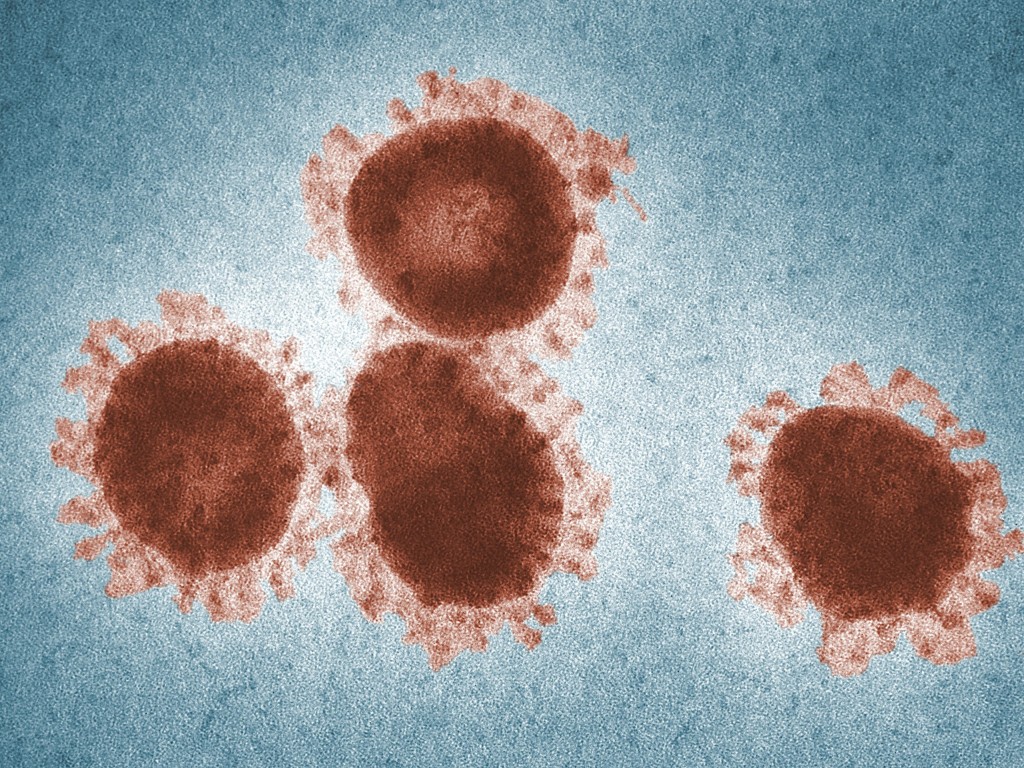
Two URV research groups have created a machine learning model that may reduce the time needed to develop drugs against new viruses.
A research team made up of members from the URV’s Asclepius and Chemoinformatics and Nutrition research groups has developed a mathematical machine-learning model that makes it possible to determine the potency of antiviral drugs. This innovative system estimates the degree of effectiveness of chemical compounds that block the reproductive capacity of the virus by interpreting three-dimensional models of its structure. If developed, this could significantly speed up the process of designing and developing anti-viral drugs and reduce the time it takes to react to future epidemics. Viruses are unable to reproduce on their own. To do so, they force the host’s healthy cells to reproduce the virus’s genetic sequence rather than their own. Virus proteases have a key role in this process: they break up polypeptide sequences to form the proteins that the virus needs to generate new individuals. They are like scissors that cut the long chains of polyproteins to form specific proteins, depending on the genetic information of each cell or virus. “It’s like a paragraph without spaces: the protease would be a word that has the capacity to separate other words so that the text makes sense,” explains Gerard Pujadas, from the Chemoinformatics and Nutrition research group. The mathematical model they have created has successfully determined which drugs can effectively prevent SARS-CoV-2 from reproducing and to what extent. This machine learning system works by encoding the three-dimensional structure of proteases and processing the data to determine which drug fits best and, therefore, best inhibits them. Without proteases, the virus cannot reproduce. All this was possible thanks to the considerable amount of information available on this virus, its proteases and how they are affected by drugs, since these models need to be “trained” to make them functional and improve their performance, says Carme Julià, a researcher in the Asclepius research group.
“The aim is not only to predict whether a drug is active against a given pathology but also to estimate the degree of efficacy,” explains Francesc Serratosa, a researcher in the Asclepius research group. This model, which has proved to be functional, could play a key role in the future when, as several experts – including researchers from the URV-IISPV Autoimmunity, Inflammation and Infection research group – point out, the proliferation of infectious diseases will be more frequent due to the globalised way of life. The automatic learning system developed by the URV researchers would significantly reduce the period of development and search for drugs against new viruses, provided that information is available to train the model. “We have designed a procedure that, with the right information, will make it possible to make a rapid prediction of the viability of drugs against new viruses,” the researchers conclude.
Reference:
Fadlallah, S., Julià, C., García-Vallvé, S., Pujadas, G., & Serratosa, F. (2023). Drug Potency Prediction of SARS-CoV-2 Main Protease Inhibitors Based on a Graph Generative Model. International Journal of Molecular Sciences, 24(10), 8779. MDPI AG. Retrieved from http://dx.doi.org/10.3390/ijms24108779