06/11/2019
An algorithm can predict how drugs will interact in 85 types of cancer
This mathematical model, developed by URV researchers, has a success rate of 75%

This mathematical model, developed by URV researchers, has a success rate of 75%
Most therapies are effective against cancer only in the short term. Tumours often develop resistance and the solution to this problem may be a combination of different drugs. However, the wide variety of medicines against cancer and the number of combinations mean that carrying out laboratory tests and clinical trials with no prior indication what the results are going to be will not be materially or economically viable. So the URV researchers took up the challenge of finding a method that could predict as reliably as possible what the interaction would be between 69 drugs in 85 different types of cancer. This challenge was set by a pharmaceutical company in the form of a competition in which 160 research centres, institutions and researchers from all over the world took part. The team from the URV finished in the top ten.
Marta Sales and Roger Guimerà, from the SEES Lab research group of the Department of Chemical Engineering, used a mathematical model of multi-layer networks that enabled numerous combinations to be made between the interactions of the drugs with the different types of cancer. On the one hand, the algorithm groups the cancers that are similar and, on the other, it has another layer made up of the medicines that behave in a similar way.
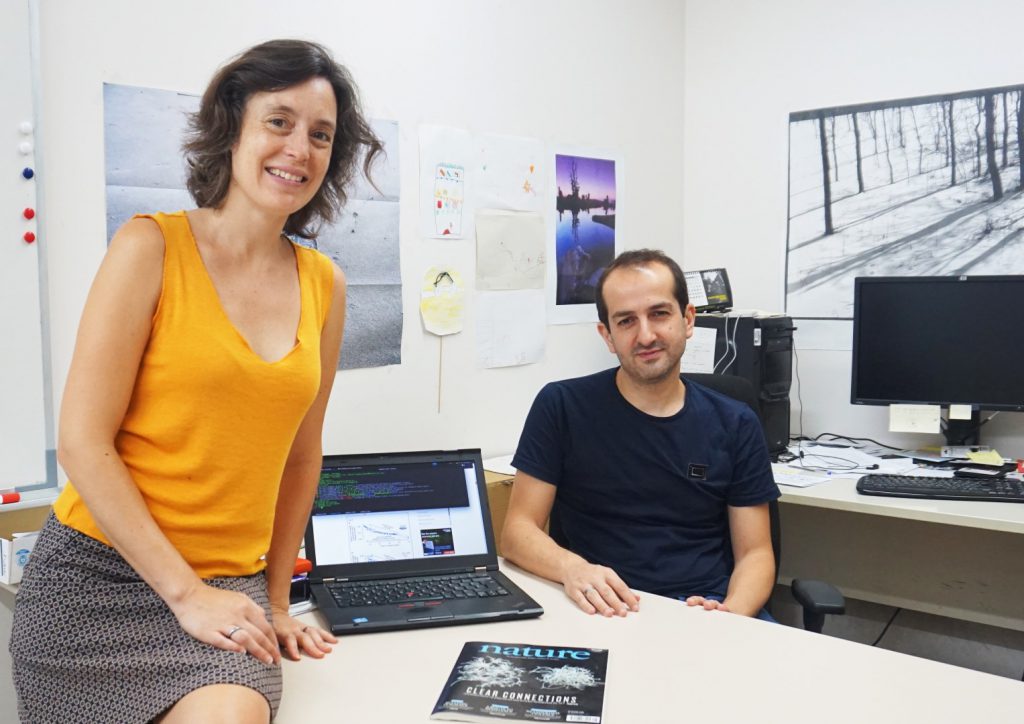
There are numerous combinations between layers and nodes, and the system makes it possible to make extremely accurate predictions about the interactions between medicines in each type of cancer. Taking into account only the known interactions, with no need for the intervention of any other biological parameter, the success rate is 75%.
“It is a very simple model, which has the added value that it is not only designed for cancer treatments but can also be used with other variables and is very straightforward,” said Roger Guimerà. All the levels can be used simultaneously which takes maximum advantage of the information contained. The same model has been used, for example, to predict if someone will like a film or not, or whether one person will decide to cooperate or compete with another.
Bibliographical reference: Community assessment to advance computational prediction of cancer drug combinations in a pharmacogenomic screen. Nature Communications (2019) 10:2674. DOI: 10.1038/s41467-019-09799-2